Survival Models in Actuarial Mathematics: Understanding the Basics
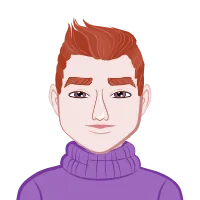
When it comes to managing risk and making informed financial decisions, actuarial mathematics plays a crucial role. Survival models, in particular, are powerful tools that help actuaries predict and analyze the likelihood of certain events occurring over time. In this blog, we'll dive into the world of survival models in actuarial mathematics, exploring their fundamental concepts, applications, and significance. Whether you're a student looking to complete your actuarial mathematics assignment or a professional seeking to deepen your understanding, this exploration will provide valuable insights into the essential aspects of survival models.
What Are Survival Models?
Survival models, also known as survival analysis or time-to-event analysis, are statistical tools used in actuarial mathematics to study the time until an event of interest occurs. These events can range from the failure of a mechanical component to the occurrence of a disease, and they play a pivotal role in assessing and managing risks in various fields, including insurance, healthcare, and finance.
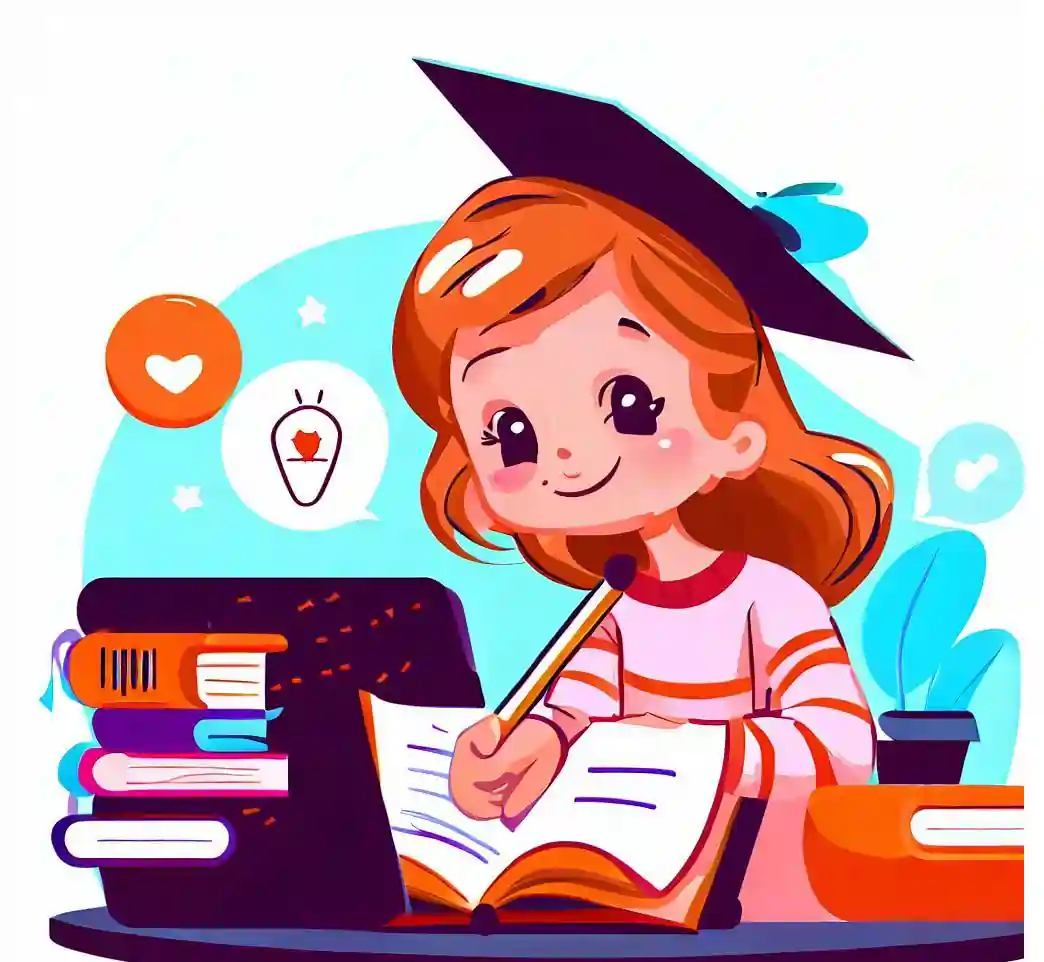
Key Components of Survival Models
Survival models are based on several essential components:
- Survival Function
- Hazard Function
-
Cumulative Hazard Function
The cumulative hazard function, denoted as Λ(t) or H(t), represents the cumulative risk of the event happening up to time t. It is derived from the hazard function and provides insights into the overall risk over a specified time interval.
The survival function, denoted as S(t), represents the probability that an event has not occurred by time t. In simple terms, it tells us the likelihood of survival up to a specific point in time. As time progresses, the survival probability decreases.
The hazard function, often denoted as λ(t) or h(t), describes the instantaneous rate at which the event of interest occurs at time t, given that it has not occurred before t. In essence, it quantifies the risk of the event happening at any given moment.
Applications of Survival Models
Survival models find wide-ranging applications in actuarial mathematics and beyond. Here are a few notable areas where these models are instrumental:
- Life Insurance
- Healthcare
- Finance
- Engineering
In the realm of life insurance, actuaries use survival models to estimate mortality rates and calculate premiums. These models help insurance companies determine the probability of policyholders passing away during a specific term, enabling them to set appropriate premium rates.
Survival analysis is extensively used in healthcare to predict patient outcomes, disease progression, and survival rates. Medical professionals rely on these models to make informed decisions regarding treatment strategies and patient care.
Survival models are applied in finance to assess the likelihood of default or bankruptcy of a company or the time until a financial instrument reaches a specific event, such as maturity or default. This information is crucial for risk management and investment decisions.
In engineering, survival models help assess the reliability and durability of components and systems. Engineers can use these models to predict when mechanical failures might occur and plan maintenance accordingly.
Types of Survival Models
Several types of survival models exist, each tailored to specific situations. Here are a few common ones:
- Kaplan-Meier Estimator
- Cox Proportional-Hazards Model
- Weibull Distribution
The Kaplan-Meier estimator is used to estimate the survival function when we have censored data. Censored data occurs when we know that an event has not occurred by a certain time but do not know if it will occur later. This estimator is particularly useful in medical research and clinical trials.
The Cox proportional-hazards model is a versatile tool that allows researchers to assess the impact of multiple variables on survival time. It assumes that the hazard function is proportional across different groups or levels of a covariate.
The Weibull distribution is often employed when modeling the failure rates of mechanical components. It provides flexibility in modeling hazard rates that increase, decrease, or remain constant over time.
Censoring in Survival Analysis
Censoring is a fundamental and intricate concept in survival analysis, significantly impacting the accuracy and reliability of the models used in actuarial mathematics and other fields. It arises when the occurrence of an event of interest is only partially observed, leading to incomplete information about the timing of events. There are three main types of censoring: right censoring, left censoring, and interval censoring, each posing unique challenges and requiring specialized techniques for analysis.
- Right Censoring
- Incomplete Information: Right censoring results in incomplete data for some subjects. While we know that the event has not occurred for them during the study period, we do not have information about whether or when it will happen in the future.
- Survival Estimation: To estimate the survival function in the presence of right censoring, researchers typically use techniques like the Kaplan-Meier estimator. This method calculates the probability of survival up to a given time point, taking into account the censored data.
- Left Censoring
- Event Occurrence Before Observation: In left censoring scenarios, events of interest may have happened prior to the study's initiation. For example, in medical research, patients may have been living with a disease before entering the study.
- Statistical Challenges: Analyzing left-censored data is complex because we need to estimate not only the survival function but also the distribution of the event times before the study. Specialized statistical methods, such as maximum likelihood estimation or EM algorithms, may be required to handle left censoring.
- Interval Censoring
- Partial Information: Interval censoring provides partial information about the event occurrence, narrowing it down to a specific time range. However, it does not pinpoint the exact time of the event.
- Applications: Interval censoring is encountered in various research scenarios, such as assessing the time it takes for a drug to relieve symptoms within a certain time frame.
- Statistical Methods: Handling interval-censored data often involves specialized statistical methods, including likelihood-based approaches, to estimate the survival function and make inferences about the event occurrence.
Right censoring is one of the most common forms of censoring in survival analysis. It occurs when we have data on the time of an event for some subjects but not for others. The key characteristic of right censoring is that the event has not yet occurred for the censored subjects by the end of the study or observation period.
Key Points:
Left censoring, in contrast, occurs when we have data on the time of an event for some subjects but do not know when the event actually occurred. This typically arises in situations where events may have occurred before the study started, leading to uncertainty about the event's timing.
Key Points:
Interval censoring is a hybrid of right and left censoring, presenting a unique challenge. In interval censoring, we only know that the event occurred within a specific time interval, but we lack precise information about the exact event time.
Key Points:
Challenges in Survival Analysis: Navigating Complexity in Actuarial Mathematics
Survival analysis is a powerful tool for understanding time-to-event data and has diverse applications in various fields. However, it comes with its own set of challenges that actuaries and researchers must grapple with. These challenges can impact the accuracy and reliability of survival models and their interpretations. Let's delve deeper into these challenges:
- Censored Data Handling
- Different Types of Censoring: Censoring can take various forms, including right censoring, left censoring, and interval censoring. Each type requires specific statistical techniques for accurate analysis. Actuaries and researchers must identify the type of censoring present in their data to apply the appropriate methods.
- Survival Function Estimation: Estimating the survival function, which represents the probability of survival up to a given time, is challenging when dealing with censored data. Methods like the Kaplan-Meier estimator are commonly used, but they can be complex to implement, especially when dealing with large datasets.
- Bias and Variance: Mishandling censored data can lead to biased survival estimates. Moreover, it can impact the precision of these estimates, resulting in wide confidence intervals that hinder the ability to make meaningful inferences.
- Model Assumptions
- Proportional Hazards Assumption: The Cox proportional-hazards model, a widely used survival model, assumes that the hazard ratios for different groups or levels of a covariate remain constant over time. Violations of this assumption can introduce bias and affect the model's predictive accuracy.
- Independence of Censoring: Another common assumption is the independence of censoring, which assumes that the probability of censoring is not related to the event of interest. In reality, this assumption may not always hold, especially in long-term studies.
- Distributional Assumptions: Some survival models assume a specific distribution for the survival times, such as the exponential or Weibull distribution. Deviations from these assumptions can lead to model misfit.
- Sample Size
- Rare Events: In certain fields, such as medical research, rare events like specific diseases or adverse outcomes can pose challenges. Obtaining a large enough sample of individuals with these rare events can be extremely difficult, affecting the statistical power of the analysis.
- Survival Analysis with Small Samples: Small sample sizes can lead to imprecise estimates and unreliable statistical inferences. Researchers often struggle to strike a balance between the availability of data and the precision of their survival models.
- Time-dependent covariates
- Dynamic Modeling: Incorporating time-dependent covariates requires more sophisticated modeling techniques, such as time-varying coefficients. These models can be computationally intensive and may necessitate advanced statistical software.
- Data Collection: Gathering and maintaining accurate and up-to-date data on time-varying covariates can be logistically challenging. Errors or gaps in data can introduce bias into the analysis.
- Interactions: Modeling interactions between time-dependent covariates adds another layer of complexity. Researchers need to carefully consider how these interactions affect survival outcomes.
Censored data is a fundamental aspect of survival analysis. It occurs when the event of interest has not occurred for some subjects by the end of the study or observation period. Effectively handling censored data is crucial, as it directly affects the estimation of survival functions and hazard rates.
Challenges:
Survival models often rely on certain assumptions about the data to simplify the modeling process. These assumptions can be critical for model selection and interpretation, but they must be carefully evaluated, as violations can lead to misleading results.
Challenges:
The adequacy of the sample size is a critical consideration in survival analysis. Obtaining a sufficient number of observations is essential to ensure the reliability of survival estimates and hypothesis testing.
Challenges:
In real-world scenarios, covariates (variables that impact survival) may change over time. This presents a complex modeling challenge, as traditional survival models assume that covariates are time-independent.
Challenges:
While survival analysis offers invaluable insights into time-to-event data, it is not without its complexities and challenges. Actuaries and researchers must navigate the intricacies of censored data, critically evaluate model assumptions, address sample size limitations, and grapple with time-dependent covariates to ensure that their survival models provide accurate and meaningful results. Meeting these challenges head-on is essential for leveraging the full potential of survival analysis in actuarial mathematics and beyond.
Future Trends in Survival Analysis
As technology and data analytics continue to evolve, survival analysis in actuarial mathematics is also advancing. Here are some future trends to watch for:
- Machine Learning Integration
- Personalized Medicine
- Big Data
Machine learning techniques, such as deep learning and random forests, are being integrated with survival analysis to improve prediction accuracy and handle complex data structures.
In healthcare, there is a growing focus on personalized medicine, and survival models will play a crucial role in tailoring treatments and interventions to individual patients based on their unique characteristics and risks.
The availability of vast amounts of data presents both opportunities and challenges in survival analysis. Actuaries are exploring ways to harness big data to enhance their modeling and risk assessment capabilities.
Conclusion
Survival models in actuarial mathematics are indispensable tools for understanding and managing risks associated with time-to-event data. From insurance to healthcare and finance to engineering, these models have diverse applications that impact decision-making and planning. While challenges exist, ongoing developments in machine learning and data analytics are poised to revolutionize the field, making survival analysis even more powerful and relevant in the years to come. As we continue to refine our understanding of survival models, we can make more informed choices, reduce uncertainty, and better navigate the complex landscape of risk.