Hypothesis Testing: Understanding the p-value
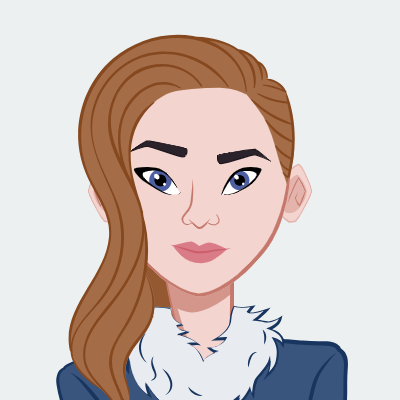
Hypothesis testing is a fundamental concept in statistics that allows us to make informed decisions based on data. It's a process through which we evaluate whether a claim about a population is supported by the evidence from a sample. Central to this process is the concept of the p-value, a critical tool for statisticians and researchers. In this comprehensive guide, we'll delve deep into hypothesis testing and explore the intricacies of the p-value, helping you complete your applied mathematics assignment.
Introduction to Hypothesis Testing
What is a Hypothesis?
At its core, hypothesis testing is about making educated guesses or assumptions, called hypotheses, about populations based on sample data. These hypotheses typically come in two forms:
- Null Hypothesis (H0): This is the default assumption that there is no effect, no difference, or no relationship between variables in the population. It represents the status quo or the commonly accepted belief.
- Alternative Hypothesis (Ha or H1): This is the statement that contradicts the null hypothesis. It suggests that there is a statistically significant effect, difference, or relationship in the population.
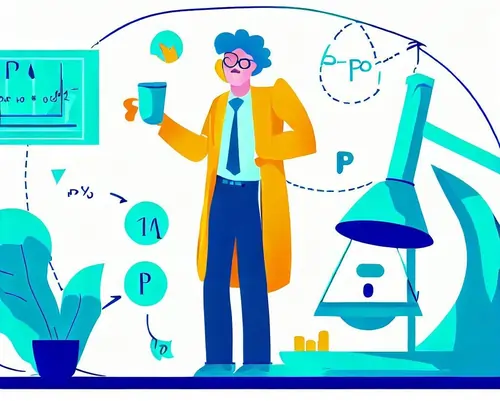
The Hypothesis Testing Process
The process of hypothesis testing involves several steps:
- Formulate Hypotheses: Clearly state the null and alternative hypotheses based on your research question and domain knowledge.
- Collect Data: Gather data through experiments, surveys, or observations.
- Select a Significance Level (α): This is the threshold for significance, often set at 0.05 or 5%. It represents the probability of making a Type I error (rejecting a true null hypothesis).
- Conduct the Test: Choose an appropriate statistical test (t-test, chi-square test, ANOVA, etc.) based on the type of data and research question. Calculate the test statistic and p-value.
- Analyze the Results: Compare the p-value to the significance level. If p ≤ α, reject the null hypothesis in favor of the alternative hypothesis. If p > α, fail to reject the null hypothesis.
- Draw Conclusions: Based on the analysis, make a decision about the population based on the sample data.
The Role of P-value in Hypothesis Testing
The p-value, short for probability value, is a crucial component of hypothesis testing. It quantifies the strength of evidence against the null hypothesis. In simple terms, it answers the question, "What is the probability of observing the data, or something more extreme, if the null hypothesis is true?"
Here are the key aspects of the p-value:
- Interpreting the p-value
- The p-value and Type I Error
- P-value Misconceptions
- The p-value is not the probability of the null hypothesis being true or false. It only provides information about the strength of evidence against the null hypothesis.
- A small p-value does not prove that the null hypothesis is false. It simply suggests that the observed data is unlikely to occur if the null hypothesis were true.
A small p-value (typically ≤ α) suggests strong evidence against the null hypothesis. This indicates that your sample data provides substantial support for the alternative hypothesis.
A large p-value (typically > α) implies weak evidence against the null hypothesis. Your sample data does not provide convincing evidence to reject the null hypothesis.
The significance level (α) is directly related to the probability of making a Type I error. When you set a low significance level (e.g., 0.05), you are being stringent and less likely to commit a Type I error. However, a low α also means that you need stronger evidence (lower p-value) to reject the null hypothesis.
It's essential to dispel common misconceptions about p-values:
Calculating the p-value
To understand how p-values are calculated, let's look at a common example using a t-test, which is used to compare means between two groups. Imagine you are studying the effectiveness of a new drug for lowering blood pressure. You have a control group and a treatment group.
The t-test Example
Null Hypothesis (H0): The new drug has no effect on lowering blood pressure, i.e., the mean blood pressure in the treatment group is equal to the mean blood pressure in the control group.
Alternative Hypothesis (Ha): The new drug is effective in lowering blood pressure, i.e., the mean blood pressure in the treatment group is different from the mean blood pressure in the control group.
Here's how the process unfolds:
- Collect Data: Measure the blood pressure in both the control and treatment groups.
- Select a Significance Level: Let's choose α = 0.05.
- Conduct the Test: Calculate the t-statistic and degrees of freedom, then find the corresponding p-value.
- Analyze the Results: Compare the p-value to α.
- Draw Conclusions: If p ≤ α, you reject the null hypothesis in favor of the alternative hypothesis.
The Mathematics of P-value Calculation
The exact calculation of the p-value depends on the statistical test used. For a t-test, the formula for calculating the t-statistic is:
t=Sample Mean−Population Mean/Standard Error of the
The t-statistic follows a t-distribution, and the p-value is determined by finding the area under the t-distribution curve that corresponds to the calculated t-statistic.
Using Software for p-value Calculation
In practice, complex statistical calculations are often done using software like R, Python, or specialized statistical packages such as SPSS or SAS. These tools simplify the process by automatically calculating the p-value and providing the results of hypothesis tests.
Interpreting p-values
The interpretation of p-values is a crucial aspect of hypothesis testing. Let's explore some scenarios to understand how to interpret p-values effectively.
Scenario 1: p ≤ α (Rejecting the Null Hypothesis)
When the calculated p-value is less than or equal to the chosen significance level (p ≤ α), you reject the null hypothesis. This implies that the observed data is inconsistent with the null hypothesis, providing evidence in favor of the alternative hypothesis.
In our blood pressure example:
- p-value ≤ 0.05 (chosen α),
- Decision: Reject the null hypothesis.
This suggests that the new drug is effective in lowering blood pressure, as the data provides strong evidence supporting the alternative hypothesis.
Scenario 2: p > α (Failing to Reject the Null Hypothesis)
When the calculated p-value is greater than the chosen significance level (p > α), you fail to reject the null hypothesis. In this case, the observed data does not provide strong enough evidence to support the alternative hypothesis.
In our blood pressure example:
- p-value > 0.05 (chosen α),
- Decision: Fail to reject the null hypothesis.
This implies that there is not enough evidence to conclude that the new drug is effective in lowering blood pressure.
Scenario 3: The Gray Area
Occasionally, the p-value may be close to the chosen significance level. For example:
- p-value ≈ 0.048 (close to α = 0.05),
- Decision: Some ambiguity.
In such cases, it's important to consider other factors, such as the practical significance of the finding and the potential consequences of Type I and Type II errors, when making a decision. The p-value alone does not dictate whether to accept or reject the null hypothesis.
Common Misinterpretations and Pitfalls
Interpreting p-values can be tricky, and there are several common misconceptions and pitfalls to avoid:
- P-value Thresholds
- "P-hacking"
- Large Sample Size and p-values
- Multiple Comparisons
Using a fixed p-value threshold (e.g., always choosing α = 0.05) can lead to incorrect conclusions. It's essential to select the significance level based on the context and consequences of the decision.
P-hacking refers to the practice of collecting and analyzing data in multiple ways until a significant result (p ≤ α) is found. This can inflate the Type I error rate and lead to false discoveries.
With a very large sample size, even tiny differences from the null hypothesis can yield significant p-values. It's important to consider the effect size (magnitude of the difference) in addition to the p-value.
When conducting multiple tests simultaneously, the probability of obtaining at least one significant result by chance increases. Techniques like Bonferroni correction are used to adjust for this.
The Role of Effect Size
While p-values provide information about statistical significance, they do not tell you about the practical significance or the size of the effect. Effect size measures how meaningful or substantial a difference or relationship is in the real world.
Common effect size measures include Cohen's d (for comparing means), odds ratios (for comparing proportions), and correlation coefficients (for assessing relationships). Understanding effect size is crucial because even a statistically significant result may not have practical relevance if the effect size is negligible.
For example, imagine a study comparing the effectiveness of two teaching methods on test scores. Method A results in a statistically significant improvement over Method B, but the effect size is tiny. In this case, while the p-value suggests a difference, it may not be practically meaningful for educators and students.
Limitations and Criticisms of p-values
While p-values are widely used and provide valuable information, they are not without their limitations and criticisms:
- Binary Decision
- Arbitrary Significance Levels
- Misinterpretation
- Publication Bias
Hypothesis testing, based on p-values, forces a binary decision: reject or fail to reject the null hypothesis. This can oversimplify complex scientific questions and ignore nuances in the data.
The choice of significance level (α) is somewhat arbitrary and can influence the results. Researchers may be tempted to adjust α after seeing the data, leading to biased conclusions.
P-values are often misunderstood or misinterpreted, leading to incorrect conclusions. This has led some statisticians and researchers to call for a shift towards more nuanced approaches to statistical inference.
Studies with significant results (p ≤ α) are more likely to be published, leading to publication bias. This can distort the scientific literature and create an inflated perception of the effectiveness of interventions.
Alternatives to p-values
Given the limitations of p-values, some researchers advocate for alternative approaches to statistical inference. These alternatives include:
- Confidence Intervals
- Bayesian Inference
- Effect Size and Practical Significance
- Open Science Practices
Confidence intervals provide a range of plausible values for a parameter (e.g., a mean) instead of a binary decision. They offer a more informative picture of the data.
Bayesian statistics incorporates prior knowledge and beliefs into the analysis, producing a posterior distribution that represents updated beliefs about the parameter of interest.
Emphasizing effect size and practical significance over p-values can help researchers focus on meaningful results rather than just statistically significant ones.
Transparent reporting of methods, data, and results can help mitigate issues like p-hacking and publication bias. Open science practices promote reproducibility and robust research.
Conclusion
The p-value is a powerful tool in hypothesis testing, providing a quantitative measure of the strength of evidence against the null hypothesis. However, it should be used with caution and in conjunction with other statistical measures and considerations. Understanding the p-value's role, interpreting it correctly, and recognizing its limitations are essential for conducting sound scientific research and making informed decisions based on data.
As the field of statistics continues to evolve, researchers are exploring alternative approaches to statistical inference that offer more nuanced and informative ways to draw conclusions from data. Whether you embrace traditional p-value-based hypothesis testing or explore these alternatives, the goal remains the same: to make robust, evidence-based decisions that advance our understanding of the world.