Unraveling the Complexity of Fractals: Calculating Fractal Dimensions
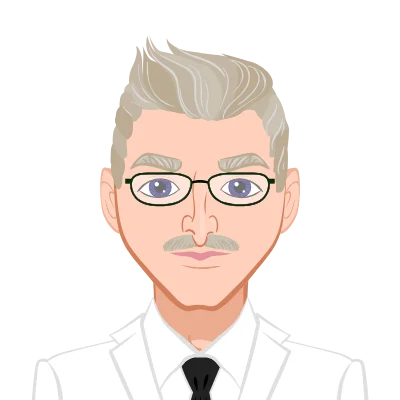
Fractals, those enigmatic geometric shapes that appear infinitely intricate, have fascinated mathematicians and scientists for decades. Their allure lies not only in their aesthetic appeal but also in their capacity to model complex, irregular patterns found in nature and various scientific disciplines. For university students grappling with math assignments, understanding how to calculate fractal dimensions is a crucial skill. In this blog post, we will embark on a theoretical journey to explore the intricacies of fractal dimension calculation and decipher what these dimensions indicate about the complexity and irregularity of fractals. So, fasten your mathematical seatbelts as we delve into the world of fractal dimensions to complete your Fractal Geometry assignment and help you solve your math assignment.
What Are Fractals?
Before diving into fractal dimension, let's first grasp the essence of fractals. Fractals are geometric shapes or patterns that exhibit self-similarity across multiple scales. In simpler terms, as you zoom in or out on a fractal, you'll notice that smaller parts of it resemble the whole structure. This property is what makes fractals both fascinating and challenging to understand.
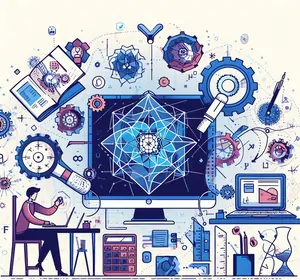
The Need for Fractal Dimensions
Fractals come in various forms, from the famous Mandelbrot set to the branching patterns of trees and the irregular coastline of a landmass. Understanding their complexity and irregularity requires a quantitative measure, and that's where fractal dimensions come into play.
Fractal dimensions are numerical values that characterize the space-filling properties of fractals. They provide a way to quantify how a fractal fills space, allowing us to differentiate between seemingly similar patterns. Two of the most common fractal dimensions are the Hausdorff-Besicovitch dimension (or simply the Hausdorff dimension) and the box-counting dimension.
Calculating the Hausdorff Dimension
The Hausdorff dimension is a fundamental concept in fractal geometry. It describes the "thickness" of a fractal in mathematical terms. The Hausdorff dimension is often calculated using a method known as the "covering dimension." Here's a simplified explanation of the process:
- Covering: Start by covering the fractal with smaller shapes (e.g., squares, circles, or rectangles) of a fixed size.
- Measure: Measure the size of the covering shapes required to cover the fractal completely.
- Scaling: Decrease the size of the covering shapes and repeat the process. Keep track of how many shapes are needed at each scale.
- Hausdorff Dimension: The Hausdorff dimension is determined by analyzing how the number of covering shapes needed scales with the size of the shapes. Specifically, it involves taking the limit as the size of the shapes approaches zero.
The Hausdorff dimension quantifies the fractal's capacity to fill space, with higher values indicating greater complexity and irregularity.
Calculating the Box-Counting Dimension
The box-counting dimension offers another perspective on the complexity of fractals. This method involves dividing the fractal into smaller boxes of a fixed size and counting how many boxes contain part of the fractal. The steps to calculate the box-counting dimension are as follows:
- Divide into Boxes: Cover the fractal with a grid of boxes, with each box having a fixed size.
- Count Boxes: Count the number of boxes that contain at least part of the fractal.
- Scaling: Decrease the size of the boxes and repeat the process. Note how the number of boxes containing the fractal scales with the size of the boxes.
- Box-Counting Dimension: The box-counting dimension is determined by analyzing how the number of boxes needed scales as the box size decreases. As with the Hausdorff dimension, the limit is taken as the box size approaches zero.
The box-counting dimension also reflects the fractal's complexity, with higher values indicating a more intricate and irregular structure.
What Fractal Dimensions Indicate
Fractal dimensions provide valuable insights into the complexity and irregularity of fractals. A higher Hausdorff or box-counting dimension signifies greater intricacy, suggesting more detailed self-similarity across scales. These dimensions serve as quantitative measures, helping us distinguish between seemingly similar patterns and understand the underlying structure of fractals in various applications.
- Hausdorff Dimension: A higher Hausdorff dimension signifies that the fractal exhibits more intricate self-similarity across different scales. It suggests that the fractal has a more complex and irregular structure. For example, the famous Mandelbrot set has a Hausdorff dimension greater than 1, reflecting its highly complex and infinitely detailed nature.
- Box-Counting Dimension: Similar to the Hausdorff dimension, a higher box-counting dimension indicates a more complex and irregular fractal. It measures how densely the fractal fills space, providing insight into its intricacy.
Applications of Fractal Dimensions
Fractal dimensions find diverse applications across scientific and practical domains. In fields like image compression, biomedical imaging, and financial markets, they enable efficient data analysis. They also aid in environmental studies, computer graphics, and natural phenomena analysis, showcasing the versatility and significance of fractal geometry in real-world scenarios.
- Image Compression: Fractal dimensions play a pivotal role in image compression. By identifying and quantifying self-similar patterns within images, they enable efficient encoding and reconstruction of complex visual data. Fractal-based compression techniques offer advantages in terms of preserving image quality while reducing file size, making them valuable in applications like digital photography, video streaming, and telemedicine. These methods not only save storage space but also enhance the speed of data transmission, a critical factor in today's digital age. The ability to harness fractal dimensions empowers professionals in diverse fields to optimize data handling and storage while maintaining high-quality visual content.
- Natural Phenomena: Natural phenomena often exhibit fractal characteristics, and understanding their fractal dimensions can shed light on complex patterns in nature. For instance, the coastline of a landmass appears jagged and irregular, but fractal analysis can help quantify its intricacies, aiding in environmental conservation and geological studies. Cloud formations and river networks also display fractal properties, making fractal dimensions a valuable tool for meteorology and hydrology. By applying fractal geometry to natural phenomena, researchers gain deeper insights into these intricate systems, helping us better comprehend the world around us and solve complex environmental challenges.
- Biomedical Imaging: Biomedical imaging relies on fractal dimensions to explore the intricacies of biological structures. In this context, fractal analysis helps decipher complex patterns in blood vessels, cellular structures, and tissue textures. By quantifying the irregularity and self-similarity within these systems, researchers gain valuable insights for diagnostics and medical research. For instance, in the study of blood vessels, fractal dimensions can reveal changes in vessel branching patterns associated with diseases, aiding in early detection and treatment. The application of fractal dimensions in biomedical imaging underscores their importance in advancing healthcare and understanding the complexity of living organisms at a microscopic level.
- Financial Markets: In financial markets, fractal dimensions play a pivotal role in understanding price dynamics and market behavior. Traders and analysts employ fractal analysis to identify patterns and trends in asset prices. Fractal dimensions provide insights into the self-similarity and complexity of price movements across different timeframes, aiding in risk assessment and decision-making. Moreover, they contribute to the development of trading strategies and models that can adapt to changing market conditions. By applying fractal dimensions, financial professionals gain a deeper understanding of market irregularities and make more informed investment choices, illustrating the practical relevance of fractal geometry in the world of finance.
- Computer Graphics: Computer graphics harness the power of fractal dimensions to create realistic and visually stunning natural landscapes, textures, and patterns. Fractal algorithms generate intricate terrain models, forests, and even complex organic structures like clouds. By incorporating fractal principles, computer graphics artists can achieve a remarkable level of detail and realism in digital environments. This application extends beyond entertainment, as industries such as architecture and urban planning benefit from the ability to simulate complex natural landscapes with ease. Fractal dimensions play a pivotal role in making virtual worlds and simulations more immersive and believable.
- Environmental Studies: In environmental studies, fractal dimensions are invaluable tools for quantifying the intricacies of natural landscapes. They help analyze the complexity of various ecological features such as forest canopies, river networks, and geological formations. Fractal analysis offers insights into the self-similar patterns found in these natural systems, aiding in habitat conservation, land management, and biodiversity assessments. By understanding the fractal dimensions of these environmental elements, researchers can make informed decisions to preserve and sustainably manage our planet's diverse ecosystems, contributing to a more holistic and responsible approach to environmental science and conservation efforts.
Challenges and Limitations
While fractal dimensions are powerful tools, they come with challenges. Different calculation methods can yield varying results, making method selection critical. The resolution of measurements affects accuracy, with finer resolutions revealing more details. Interpreting high dimensions isn't always straightforward, requiring context to understand their real-world implications and limitations. Some important considerations include:
- Choice of Method: Choice of method in calculating fractal dimensions is pivotal. Various techniques such as box-counting, correlation dimension, and information dimension can produce different outcomes for the same fractal object. Selecting the appropriate method depends on the specific characteristics of the fractal and the research goals. A method that captures the intricacies of a highly irregular fractal may not be suitable for a more regular one. This makes method selection a nuanced process, requiring an understanding of the fractal's properties and the precision needed for the analysis. Researchers must be diligent in their choice to ensure that the calculated fractal dimension accurately represents the underlying structure.
- Resolution: Resolution plays a crucial role in the accurate calculation of fractal dimensions. It refers to the level of detail captured in measurements, such as grid size or sampling intervals. Finer resolutions reveal more intricate features of fractals, providing a comprehensive view of their complexity. However, excessively high resolutions can lead to computational challenges and increased data requirements. Striking the right balance between resolution and computational feasibility is essential when working with fractals. Researchers must carefully consider the resolution choice to ensure that fractal dimension calculations capture the relevant aspects of a fractal's structure without overwhelming the analysis with unnecessary data or computational complexity.
- Interpretation: Interpreting fractal dimensions is a nuanced task. A high dimension value doesn't always equate to greater complexity in a meaningful sense. For instance, a mathematical construct with a high fractal dimension may not necessarily exhibit intricate or interesting self-similarity when applied to real-world phenomena. Context is crucial; a high dimension could indicate redundancy in the data, obscuring genuine patterns. Moreover, a low fractal dimension doesn't always signify simplicity; it could result from measurement limitations or the specific method used. Therefore, while fractal dimensions offer valuable insights, their interpretation should consider the unique characteristics and applications of the fractal being analyzed, maintaining a cautious and context-sensitive approach.
Conclusion
In conclusion, understanding how to calculate fractal dimensions is a valuable skill for university students studying mathematics and related disciplines. These dimensions provide quantitative insights into the complexity and irregularity of fractals, helping us distinguish between seemingly similar patterns. The Hausdorff dimension and box-counting dimension offer different perspectives on the same concept, allowing for a comprehensive analysis of fractal structures. As you embark on your math assignments involving fractals, remember that these dimensions are not mere mathematical abstractions but tools with real-world applications in fields ranging from art and science to finance and environmental studies. By mastering the calculation and interpretation of fractal dimensions, you'll be better equipped to solve your math assignments and explore the fascinating world of fractal geometry.