Necessary Topics to Master Before Completing Statistical Modeling Assignments
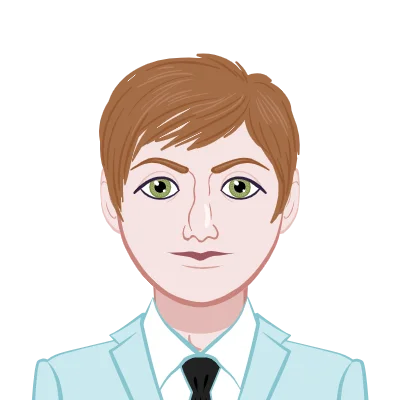
Honing the Foundation
Before delving into statistical modeling assignments, it's essential to have a solid grasp of the foundational concepts. A strong understanding of probability, distributions, descriptive statistics, hypothesis testing, regression analysis, experimental design, and model validation sets the stage for successful statistical modeling assignments. These foundational topics provide the tools to unravel data intricacies and create robust models that extract meaningful insights from complex datasets. Here are the key topics you should be well-versed in:
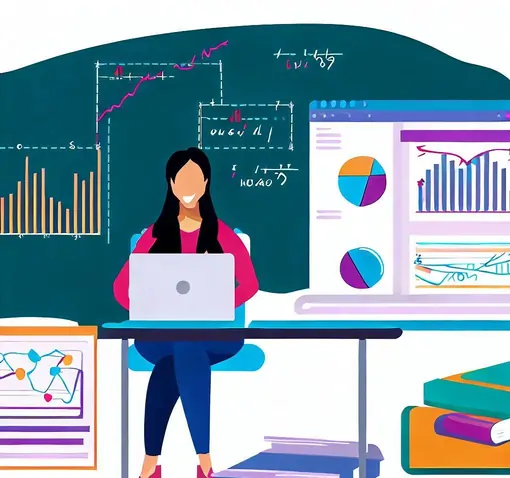
- Probability and Distributions
- Descriptive Statistics
- Hypothesis Testing and Inference
- Regression Analysis
- Experimental Design
- Model Validation and Overfitting
- Understand the Assignment
- Data Exploration
- Choose the Right Model
- Feature Selection and Engineering
- Model Building and Training
- Model Evaluation
- Iterate and Optimize
- Practice Regularly
- Seek Resources
- Collaborate and Discuss
- Learn from Feedback
- Real-World Applications
Probability forms the bedrock of statistical modeling, enabling us to quantify uncertainty. It's the language through which we express chances and make informed decisions in the face of randomness. Understanding random variables and their distributions is crucial; they're the building blocks of various modeling techniques. Distributions like the normal, binomial, and Poisson distributions help us comprehend how data is spread out and the likelihood of different outcomes.
As you embark on statistical modeling assignments, grasp the significance of these concepts. Probability and distributions offer the lens through which you view data's underlying patterns. Armed with this knowledge, you can effectively assess uncertainty, formulate hypotheses, and develop models that capture the essence of real-world phenomena. A solid foundation here paves the way for more advanced modeling techniques and a deeper understanding of data's hidden stories.
Descriptive statistics breathes life into raw data, revealing its story in a comprehensible manner. By exploring measures of central tendency like the mean, median, and mode, you gain insights into the data's typical behavior. Variability measures such as the range, variance, and standard deviation highlight data's spread and consistency.
In statistical modeling assignments, these tools are your initial glimpse into the dataset's characteristics. They aid in identifying potential outliers, understanding data distribution, and making informed decisions on preprocessing steps. For effective communication, visualizations like histograms, box plots, and scatter plots paint a clearer picture of the data's landscape.
Incorporate descriptive statistics into your analytical toolkit. They empower you to interpret data, discern patterns, and ultimately construct accurate models. A strong grasp of these fundamentals enhances your ability to extract meaningful insights from the often intricate world of statistical modeling.
Hypothesis testing and inference are the bridges connecting sample data to broader conclusions. They provide a systematic way to make sense of observations and draw reliable insights. In statistical modeling, hypothesis testing involves proposing assumptions about populations and then assessing the likelihood of those assumptions based on sample data.
These concepts guide your decision-making process in assignments. By setting up null and alternative hypotheses, calculating p-values, and establishing confidence intervals, you're equipped to quantify the uncertainty associated with your findings. These techniques ensure your results are not merely based on chance but hold true significance.
Mastering hypothesis testing and inference is akin to becoming a detective of data. These tools enable you to uncover hidden relationships, validate assumptions, and communicate your findings with confidence. In the realm of statistical modeling, these skills empower you to transform raw data into actionable insights.
Regression analysis is the flashlight illuminating the intricate relationships between variables. It's a versatile tool that helps you understand how one variable influences another. Linear regression, multiple regression, and logistic regression are essential techniques that model these connections.
In assignments, regression analysis plays a pivotal role in predictive modeling. By identifying the underlying patterns and quantifying relationships, you can make informed forecasts and decisions. Understanding coefficients, interpreting results, and assessing model fit are crucial steps in this process.
Equipped with regression analysis skills, you can unveil insights hidden within the data's complexity. These insights inform strategies, policies, and business decisions across various domains. By mastering regression, you position yourself to solve real-world problems and extract valuable knowledge from data.
Experimental design is the compass guiding you through the realm of data collection and analysis. It's the meticulous planning that ensures your findings are credible and meaningful. Understanding the distinction between observational and experimental studies is crucial. By carefully controlling variables, randomizing conditions, and considering potential confounding factors, you reduce biases and enhance the reliability of your results.
In assignments, a solid grasp of experimental design helps you create a robust foundation for analysis. Whether you're investigating the effects of a new drug, evaluating marketing strategies, or studying social behaviors, proper experimental design ensures your findings accurately reflect reality.
By mastering experimental design, you cultivate the ability to set up controlled studies that provide insights with integrity. This skill is invaluable in the world of statistical modeling, enabling you to contribute to the pool of accurate knowledge and make informed decisions based on sound evidence.
Model validation is the compass steering you toward accurate predictions and reliable insights. It's the process of assessing how well your model performs on unseen data. However, a pitfall to watch out for is overfitting, where a model fits training data too closely and fails to generalize well to new data.
In assignments, model validation is your litmus test for success. Techniques like cross-validation ensure your model doesn't suffer from overfitting. By splitting data into training and validation sets, you're able to fine-tune your model's parameters and evaluate its performance on fresh data.
Understanding model validation and guarding against overfitting is crucial in statistical modeling. It ensures your insights are not artifacts of your training data but rather robust representations of reality. By mastering these aspects, you position yourself to develop models that stand up to the complexities of real-world data.
Approaching Statistical Modeling Assignments
Once you've solidified your understanding of the foundational topics, it's time to tackle statistical modeling assignments with confidence. Here's a step-by-step guide to help you navigate these assignments effectively:
The first step to acing a statistical modeling assignment is deciphering the task at hand. Carefully read the instructions to grasp the objectives, dataset, and required techniques. Clarity is paramount. Seek clarification if needed. Understanding the assignment ensures you channel your efforts in the right direction from the start, setting the stage for a well-executed and relevant analysis that aligns seamlessly with the assignment's requirements.
Before embarking on any modeling journey, data exploration is your compass. It's the process of unearthing insights, patterns, and anomalies within your dataset. By visualizing distributions, identifying outliers, and understanding variable relationships, you lay the groundwork for informed decisions throughout your assignment. Data exploration not only helps you clean and preprocess data effectively but also guides your modeling choices. It's the initial step that sets the tone for a successful statistical modeling endeavor.
Selecting the appropriate model is akin to choosing the right tool for a task. Just as a carpenter wouldn't use a hammer to paint a canvas, you shouldn't use a model that doesn't fit your data. In assignments, understanding the problem's nature—regression, classification, clustering—guides your choice. Picking the right model increases the chances of obtaining accurate and meaningful results, setting the stage for successful statistical modeling.
Feature selection and engineering are the chisels you use to craft models that capture data's essence. In assignments, identifying the right features to include can significantly impact your model's accuracy. Meanwhile, feature engineering involves transforming or creating variables to better represent relationships.
By understanding the relevance of features and applying creative engineering, you enhance your model's ability to discern patterns and make accurate predictions. These skills empower you to extract maximum value from data, shaping it into a form that magnifies the power of statistical modeling.
Model building is the art of transforming data into predictive power. Assignments challenge you to apply theoretical knowledge to construct models tailored to specific problems. This process involves selecting appropriate algorithms, setting hyperparameters, and training the model on the data. Through iterations and fine-tuning, you cultivate the ability to develop models that capture data intricacies and generate accurate predictions. This hands-on experience equips you to bridge the gap between theory and practical application, a crucial skill in the realm of statistical modeling.
Model evaluation is the yardstick by which you measure your model's effectiveness. It involves using specific metrics to assess how well your model performs its intended task. For regression, mean squared error quantifies prediction accuracy; for classification, metrics like accuracy, precision, and recall provide nuanced insights. By mastering model evaluation, you gain the ability to objectively determine your model's strengths and weaknesses, refining your approach and delivering more accurate results in statistical modeling assignments.
Embrace the iterative nature of statistical modeling assignments. Rarely does a model perform perfectly on the first try. Take a systematic approach to refining your work. Tweak parameters, experiment with different algorithms, and reevaluate your data preprocessing steps. Each iteration hones your model's accuracy and enhances your problem-solving skills. Through persistence and adaptation, you'll transform initial concepts into sophisticated models that extract valuable insights from complex data landscapes.
Enhancing Your Skill Set
Becoming proficient in statistical modeling takes practice and dedication. Here are additional strategies to enhance your skills and excel in your assignments:
Statistical modeling proficiency is honed through consistent practice. Engage with diverse datasets, experiment with various techniques, and tackle a range of problems. Just as athletes train to excel, regular practice builds your intuition and refines your analytical prowess. Each modeling encounter adds to your expertise, bringing you closer to mastering the art of turning data into actionable intelligence.
Expand your knowledge by tapping into a wealth of online resources. Platforms like Khan Academy, Coursera, and DataCamp offer comprehensive courses on statistical modeling. These resources provide interactive learning experiences, allowing you to grasp complex concepts at your own pace. Supplementing your coursework with these resources empowers you to dive deeper into the world of statistical modeling, fostering a strong foundation for assignments.
Engaging in discussions with peers and mentors enriches your understanding of statistical modeling. Explaining your thoughts and learning from others' viewpoints widens your horizons. Collaborative environments foster creativity and expose you to alternative approaches. Through shared insights, you can refine your strategies and conquer challenges more effectively, ultimately elevating your ability to tackle statistical modeling assignments with confidence.
Feedback on your assignments is a treasure trove of learning. Understand where you went wrong, why certain approaches were ineffective, and how to rectify mistakes. Adapting based on feedback sharpens your analytical acumen, enabling you to tackle future challenges with improved strategies and a deeper understanding of statistical modeling nuances.
Understanding statistical modeling isn't just theoretical—it has tangible real-world applications. Analyzing case studies, research papers, or business reports showcases how statistical models influence decision-making. Whether it's predicting market trends, optimizing supply chains, or studying disease spread, these models empower you to tackle complex challenges and contribute meaningfully to diverse industries, bridging the gap between data and impactful solutions.
Conclusion
A strong foundation in probability, distributions, descriptive statistics, hypothesis testing, regression analysis, experimental design, and model validation is paramount. Armed with these essential topics and guided by effective strategies, you're well-equipped to solve your statistical modeling assignments with confidence. Remember, practice and persistence are key. By mastering these concepts, you'll not only excel in your assignments but also unlock the power to solve intricate problems and extract meaningful insights from data.