Understanding Reliability Analysis: Key Topics and How to Excel in Assignments
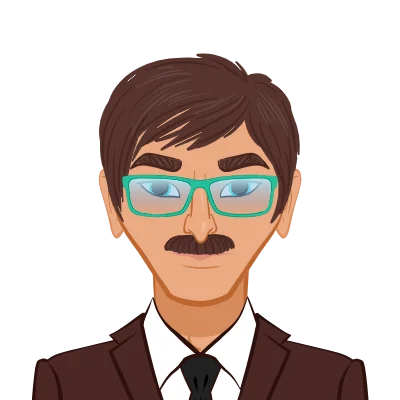
Introduction to Reliability Analysis
Reliability analysis is the cornerstone of ensuring the durability and dependability of systems, products, and processes. It's the process of assessing the probability that a system will perform its intended function without failure for a specific period under defined conditions. Whether you're dealing with mechanical systems, electronic components, software applications, or industrial processes, reliability analysis offers a structured approach to evaluate and enhance the reliability of these entities.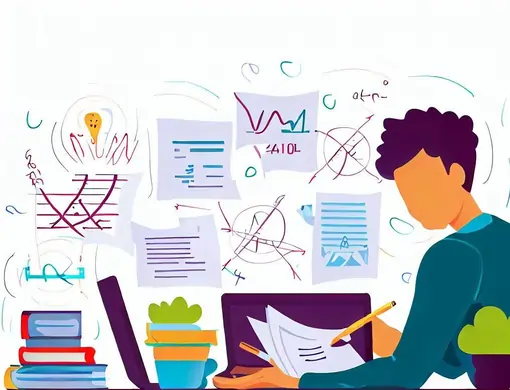
Essential Topics to Understand before Doing Reliability Analysis Assignments
Before diving into a reliability analysis assignment, it's crucial to have a solid understanding of several key topics to ensure your success in tackling the assignment effectively. Here are the essential topics you should be familiar with before starting a reliability analysis assignment:
- Basic Probability and Statistics:
- Failure Modes and Mechanisms:
- Reliability Metrics:
- Probability Distributions:
- Data Collection and Analysis:
- Reliability Improvement Techniques:
- System Reliability vs. Component Reliability:
- Software Tools and Statistical Software:
- Risk Assessment:
- Documentation and Reporting:
A strong grasp of basic probability and statistics forms the cornerstone of effective reliability analysis. Probability concepts, such as likelihood and uncertainty, provide a framework for understanding the randomness inherent in system behaviors. Statistical measures like mean, variance, and standard deviation enable us to quantify and describe variability in data. These tools are essential for modeling and analyzing failure patterns, estimating reliability metrics, and making informed decisions based on data-driven insights.
Whether you're fitting data to distributions, calculating probabilities of failure, or performing hypothesis tests, the knowledge of probability and statistics empowers you to interpret and predict system behavior accurately. In reliability analysis, this foundation ensures that your assessments are rigorous, enabling you to identify potential risks, improve system reliability, and contribute meaningfully to engineering and quality management.
Understanding failure modes and mechanisms is pivotal in reliability analysis. Every system or component has specific ways in which it can fail due to factors like wear, stress, corrosion, and fatigue. By comprehending these failure mechanisms, you can select appropriate probability distributions and models that mirror the real-world behavior of the system. Identifying potential failure modes aids in data collection and analysis, as well as in designing effective tests and experiments to gather relevant data. Moreover, this knowledge empowers you to recommend targeted improvements to enhance reliability. Whether you're dealing with mechanical, electrical, or software systems, recognizing failure modes equips you to predict and prevent failures, contributing to safer, more robust designs and operations.
Understanding key reliability metrics is paramount in assessing the performance and durability of systems. Metrics like Mean Time Between Failures (MTBF), Failure Rate (λ), and Reliability Function (R(t)) provide quantitative insights into system behavior over time. MTBF offers a clear indicator of the average operational time between failures, helping to gauge system downtime. Failure Rate quantifies the rate at which failures occur, aiding in predicting failure occurrences for maintenance planning.
The Reliability Function illustrates the probability that a system will operate without failure until a specified time, facilitating risk evaluation. These metrics enable professionals to make informed decisions about system design, maintenance strategies, and overall performance enhancement. Mastery of these metrics empowers analysts to identify critical components, anticipate potential issues, and devise strategies to optimize reliability in diverse industries, from aerospace to manufacturing.
Probability distributions serve as the building blocks of reliability analysis, providing mathematical representations of how failures occur over time. Each distribution captures specific failure patterns, allowing you to model diverse systems accurately. The exponential distribution, for instance, is suitable for components with constant failure rates, while the Weibull distribution accommodates both early-life failures and wear-out periods.
Understanding these distributions aids in estimating probabilities of failure at different time intervals, enabling you to predict system reliability and plan maintenance effectively. Proficiency in selecting and applying distributions empowers you to identify critical components, evaluate failure risks, and devise strategies for optimal system performance. By mastering probability distributions, you equip yourself with a powerful toolset to unravel complex failure behaviors and contribute decisively to reliability assessment and enhancement efforts.
Effective data collection and analysis are the cornerstones of reliable insights in any reliability analysis assignment. Skillful data collection involves identifying relevant variables, ensuring data accuracy, and minimizing bias. Once collected, data needs meticulous analysis, including cleaning, transforming, and fitting to appropriate probability distributions. Statistical techniques, like parameter estimation and hypothesis testing, unravel hidden patterns and provide a basis for making informed reliability assessments.
Proficiency in data analysis tools and software enables you to derive meaningful metrics like MTBF, failure rates, and reliability functions. Sound data practices foster robust conclusions, ensuring your reliability analysis accurately reflects the system's behavior. Whether you're deciphering failure patterns or recommending improvements, the quality of your data collection and analysis directly impacts the credibility and validity of your reliability analysis outcomes.
Understanding reliability improvement techniques is pivotal for enhancing system dependability. These strategies encompass redundancy, fault tolerance, preventive maintenance, and design modifications. Redundancy involves duplicating critical components to ensure system functionality even if one fails. Fault tolerance equips systems to continue functioning despite minor failures. Preventive maintenance minimizes unexpected breakdowns through scheduled inspections and repairs. Design modifications, informed by reliability analysis, address weak points and vulnerabilities in the system.
By mastering these techniques, you empower yourself to design and maintain systems that exhibit robustness, resilience, and longevity. Reliability improvement strategies mitigate the impact of failures, reduce downtime, and enhance overall system performance. This knowledge equips you to contribute actively to creating more reliable systems, vital across industries where dependability is paramount, from aerospace to healthcare and beyond.
Distinguishing between system reliability and component reliability is crucial for a comprehensive understanding of reliability analysis. While component reliability focuses on the failure characteristics of individual parts, system reliability considers how these components interact to influence the overall system performance. Analyzing system reliability involves assessing the impact of dependencies, interactions, and redundancies among components. This knowledge aids in identifying weak points within the system that might compromise its overall functionality.
By recognizing the intricate relationship between component and system reliability, engineers can make informed design decisions, implement effective maintenance strategies, and enhance the overall reliability of complex systems. Balancing both aspects ensures a holistic approach to reliability analysis, leading to systems that not only consist of dependable components but also work cohesively to achieve desired levels of reliability and performance.
Proficiency in software tools and statistical software is indispensable for efficient reliability analysis. Modern tools like MATLAB, Python with libraries like SciPy and NumPy, R, or specialized reliability software streamline complex calculations, data manipulation, and visualization. These tools empower you to handle large datasets, fit distributions, perform Monte Carlo simulations, and create insightful visualizations, all of which are essential for accurate reliability assessments. Leveraging these software resources not only enhances your problem-solving capabilities but also allows you to focus on the analytical aspects of the assignment rather than getting bogged down by manual calculations. Mastery of these tools equips you to navigate the intricacies of reliability analysis effectively and produce results that are both reliable and actionable within various engineering and industrial contexts.
Incorporating risk assessment into reliability analysis adds a layer of strategic planning. Identifying critical components prone to failure allows for targeted intervention. Evaluating potential consequences of failures aids in prioritizing mitigation efforts. By understanding the impact of failures on system functionality, safety, and costs, you can allocate resources effectively. This anticipatory approach enables proactive measures such as redundancy implementation or maintenance protocols to minimize downtime and mitigate adverse effects. Ultimately, integrating risk assessment into reliability analysis fosters a holistic understanding of system vulnerabilities, guiding decisions that optimize reliability, safety, and overall operational efficiency.
Effective documentation and reporting are pivotal in ensuring the value of your reliability analysis is realized. A well-structured report should encompass the entire analysis process, from data collection and methodology selection to results interpretation and recommendations. Clear and concise communication ensures that stakeholders, including colleagues, management, and clients, can understand the findings and insights without confusion. Accurate documentation not only facilitates transparency but also lays the groundwork for future analysis, making it easier to replicate or build upon your work. Furthermore, a detailed report becomes a valuable reference for decision-making, allowing stakeholders to make informed choices regarding system improvements, resource allocation, and risk mitigation strategies. Mastering this aspect of reliability analysis amplifies the impact of your work and contributes to efficient and informed decision-making across various industries.
Solving Reliability Analysis Assignments: A Step-by-Step Approach
Approach reliability analysis assignments systematically by understanding the problem, gathering relevant data, identifying appropriate reliability metrics, applying suitable probability distributions, analyzing data using statistical tools, interpreting results, and proposing actionable recommendations. This structured methodology ensures robust and insightful conclusions in your assignments. Solving assignments related to reliability analysis requires a systematic approach:
- Understand the Problem: Begin by thoroughly comprehending the assignment's requirements, system characteristics, and objectives. Identify key concepts, metrics, and data needed for the analysis. This step forms the foundation for accurate decision-making throughout the reliability analysis process, ensuring that your approach aligns with the assignment's scope and goals.
- Gather Data: Collecting accurate and comprehensive data is pivotal in reliability analysis. This involves identifying relevant variables, selecting appropriate data sources, and ensuring data quality. Reliable data forms the foundation for informed decision-making and meaningful analysis, making meticulous data gathering a crucial initial step in the process.
- Identify the Reliability Metrics: Selecting the right reliability metrics is pivotal. Depending on the problem, determine whether Mean Time Between Failures (MTBF), Failure Rate (λ), or Reliability Function (R(t)) is relevant. This choice guides subsequent analysis, offering insights into failure frequency, probability, and overall system performance.
- Choose Appropriate Distributions: Selecting the right probability distribution is pivotal in reliability analysis. Each distribution models different failure patterns. For instance, the exponential distribution suits random failures, while the Weibull distribution accounts for wear-out failures. An informed choice guarantees accurate predictions and insights into how systems behave over time, bolstering the credibility of your analysis.
- Apply Statistical Tools: Employ statistical tools to analyze data, estimate parameters of probability distributions, and calculate essential reliability metrics. Tools like MATLAB, Python's statistical libraries, and specialized software enable accurate modeling, facilitating data-driven decisions for reliability assessments. Proficiency in these tools ensures precise and reliable results in your analysis process.
- Interpret Results: Interpreting the outcomes of your reliability analysis is the bridge between raw data and meaningful insights. Analyze reliability metrics, probability distributions, and failure patterns to understand the system's behavior. Assess whether the system meets desired reliability standards, identify weak points, and suggest strategies for improvement based on your findings, contributing to effective decision-making.
- Provide Recommendations: After conducting a comprehensive reliability analysis, offering well-grounded recommendations becomes pivotal. Drawing from your insights into failure patterns and system behavior, suggest strategies for enhancing reliability. These recommendations might include maintenance schedules, component improvements, or system design modifications, all aimed at ensuring sustained performance and minimizing failures.
Conclusion
Reliability analysis is a crucial aspect of engineering and quality management. By grasping the core topics such as probability, reliability metrics, failure distributions, and improvement strategies, you can lay a solid foundation for effective reliability analysis. When approaching assignments in this field, a step-by-step approach involving problem understanding, data analysis, and interpretation will guide you towards accurate and insightful conclusions. As you delve deeper into the intricacies of reliability analysis, you'll find yourself equipped with the skills to ensure the dependability and longevity of various systems and processes.