A Step-by-step Guide on Solving Key Topics Tested in Multivariate Analysis Assignments
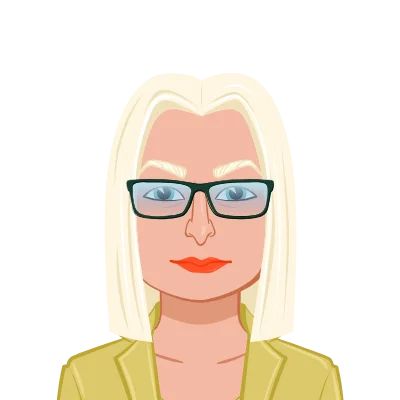
Understanding the Basics of Multivariate Analysis
Mastering the basics of multivariate analysis is crucial before delving into assignments. Learn to differentiate between variable types, comprehend correlations, and grasp the concept of Principal Component Analysis (PCA). This foundation will empower you to dissect complex relationships among variables and lay the groundwork for more advanced techniques.
.webp)
Multivariate analysis is a powerful statistical approach that deals with the simultaneous analysis of multiple variables to understand complex relationships and patterns within data. It is extensively used in various fields such as economics, social sciences, biology, and marketing. If you're about to embark on an assignment on multivariate analysis, there are several crucial topics you should be well-acquainted with to ensure your success. In this blog, we'll delve into these essential topics and provide you with effective strategies to conquer your multivariate analysis assignments.
- Variables and Data Types
- Correlation and Covariance
- Principal Component Analysis (PCA)
Variables form the cornerstone of any multivariate analysis. They can be continuous, categorical, or ordinal, each holding unique significance in analysis. Continuous variables, such as age or income, provide numerical data and demand appropriate statistical methods like regression. Categorical variables, like gender or region, require techniques such as chi-squared tests to reveal associations. Ordinal variables, such as education levels, involve ordered categories and demand specialized attention for accurate interpretation. Understanding these distinctions is essential as they determine the tools and techniques you'll employ in your analysis. A firm grasp of variable types equips you to choose the right path for uncovering insights within complex datasets, ensuring that your assignment stands on a solid analytical foundation.
Correlation and covariance serve as fundamental building blocks in multivariate analysis. Correlation measures the strength and direction of the linear relationship between two variables, while covariance quantifies the degree to which two variables vary together. Understanding these concepts is essential for deciphering the interdependencies among variables, which forms the basis of more intricate analyses. A positive covariance indicates that variables tend to increase or decrease together, while negative covariance suggests an inverse relationship. However, covariance's scale makes interpretation challenging. Correlation, on the other hand, scales covariance down to a range between -1 and 1, providing a more intuitive measure of association. Proficiency in interpreting and calculating correlation and covariance is crucial for unlocking insights from your multivariate data.
Principal Component Analysis (PCA) is a transformative technique in multivariate analysis. It's used to simplify complex datasets by converting correlated variables into a new set of orthogonal (uncorrelated) variables called principal components. These components are ranked by the amount of variance they explain in the original data. By doing so, PCA reduces the dimensionality of the data while retaining as much of the original information as possible.
PCA is widely employed in various fields, including image processing, genetics, and finance, to enhance data visualization, noise reduction, and pattern recognition. Understanding PCA's mathematical underpinnings, interpreting eigenvalues and eigenvectors, and knowing how to decide on the number of components to retain are pivotal skills when working with this technique. Mastery of PCA equips you with a powerful tool to reveal hidden structures within data and make more informed decisions in various analytical scenarios.
Selecting the Right Multivariate Analysis Techniques
Choosing the appropriate multivariate analysis technique is a pivotal step in data exploration. Factor Analysis helps uncover underlying patterns in observed variables, while Cluster Analysis groups similar data points for deeper insight. Multivariate Analysis of Variance (MANOVA) extends ANOVA to multiple dependent variables. Understanding these techniques' strengths and when to apply them ensures your analysis is tailored to the complexity of your dataset.
- Factor Analysis
- Cluster Analysis
- Multivariate Analysis of Variance (MANOVA)
Factor Analysis is a significant multivariate analysis technique used to explore the underlying structure of complex datasets. It identifies latent factors that influence observed variables, helping to simplify data interpretation. By grouping variables into factors, Factor Analysis reduces dimensionality while retaining essential information. Interpreting factor loadings provides insights into which variables are strongly related to each factor.
This technique is widely applied in psychology, marketing, and social sciences to uncover hidden constructs influencing observed behaviors. Understanding the types of factor models, such as exploratory and confirmatory factor analysis, and their applications, empowers analysts to uncover meaningful patterns within data. Factor Analysis demands careful consideration of model fit and a deep grasp of data domains, making it a powerful tool to unravel intricate relationships and simplify complex information structures.
Cluster Analysis is a significant multivariate analysis technique that categorizes similar data points into distinct groups or clusters. This technique is particularly useful in identifying patterns, relationships, and structures within data that might not be apparent initially. By grouping similar observations, you can uncover insights, segment customer profiles, or classify data into meaningful categories. Understanding different clustering algorithms, such as K-means or hierarchical clustering, and selecting the appropriate one based on your data's characteristics is essential. Evaluating the quality of clusters and deciding on the optimal number of clusters demands both technical prowess and an analytical mindset. In your assignment, effectively applying cluster analysis can reveal valuable information and patterns that might drive critical decision-making in various fields, from marketing strategies to medical research.
Multivariate Analysis of Variance (MANOVA) is a robust statistical method that extends the principles of Analysis of Variance (ANOVA) to situations involving multiple dependent variables. MANOVA allows researchers to examine how several independent variables simultaneously influence two or more related dependent variables. By considering the correlations between dependent variables, MANOVA provides a more comprehensive understanding of the underlying relationships within the data.
Researchers use MANOVA to determine if groups differ significantly across the combined dependent variables, while controlling for the possibility of Type I errors that can occur when analyzing each dependent variable separately. Interpretation of MANOVA results involves understanding the Pillai's Trace, Wilks' Lambda, Hotelling's Trace, and Roy's Largest Root statistics, among others.
Utilizing MANOVA effectively requires careful consideration of assumptions, such as multivariate normality and homogeneity of variance-covariance matrices. By incorporating MANOVA into your multivariate analysis toolkit, you can gain deeper insights into complex relationships and enhance your ability to draw meaningful conclusions from multidimensional data.
Data Preprocessing and Assumptions
Before embarking on multivariate analysis, ensure your data is primed for accurate results. Cleanse your data of outliers and missing values, as these can skew your findings. Additionally, validate assumptions such as normality and homoscedasticity, ensuring your data aligns with the requirements of your chosen analysis techniques. Proper preprocessing safeguards the integrity of your analysis and the reliability of your conclusions. Before applying any multivariate analysis techniques, ensure your data is properly preprocessed and assumptions are met:
- Data Cleaning
- Normality and Homoscedasticity
Data cleaning is an indispensable initial step in multivariate analysis. It involves identifying and rectifying inconsistencies, outliers, and missing values within your dataset. Outliers can skew results, while missing values can introduce bias. Imputation techniques like mean substitution or regression can help address missing data. Moreover, maintaining uniformity in measurement units and formats across variables ensures accurate analysis.
Through data cleaning, you enhance the reliability and validity of your analysis. It minimizes the risk of drawing erroneous conclusions based on flawed data. Rigorous data cleaning also prepares you for subsequent steps, like exploratory analysis and modeling. Remember that transparency in documenting the cleaning process is essential for both reproducibility and addressing any potential skepticism about the quality of your data.
Two crucial assumptions in multivariate analysis are normality and homoscedasticity. Normality assumes that the variables are distributed normally within each group or condition, impacting the validity of statistical tests. To assess normality, use techniques like histograms, Q-Q plots, and Shapiro-Wilk tests. Levene's test or Bartlett's test can help you check homoscedasticity. If these assumptions aren't met, transformations or robust techniques might be necessary. Ensuring your data adheres to these assumptions enhances the reliability of your multivariate analysis results and strengthens the validity of your conclusions.
Interpreting Results and Drawing Conclusions
Interpreting multivariate analysis results requires a keen understanding of the techniques used and their implications. Unravel eigenvalues' significance in PCA, and grasp the insights biplot analysis offers. By effectively translating these outcomes, you can uncover intricate relationships and glean meaningful insights from complex data patterns. Effectively interpreting the results of your multivariate analysis is a pivotal aspect of your assignment. Here's how to do it:
- Eigenvalues and Explained Variance
- Biplot Analysis
In multivariate analysis, eigenvalues play a pivotal role, particularly in techniques like Principal Component Analysis (PCA). Eigenvalues quantify the amount of variance captured by each principal component, offering insight into their importance. Higher eigenvalues indicate components that retain more original data variability. Calculating the proportion of total variance explained by each eigenvalue helps determine the number of principal components to retain. This balance between reducing dimensionality and retaining significant variance is essential for effective data reduction and interpretation, enabling you to focus on the most informative aspects of your data.
Biplot analysis is a visual tool that combines information from both variables and observations into a single graph. It allows you to represent high-dimensional data in a two-dimensional space, where each point represents an observation and vectors represent variables. This technique provides a holistic view of relationships between variables and observations, facilitating the identification of clusters, trends, and correlations. By comprehending the arrangement of points and vectors on the biplot, you can unveil hidden patterns, make comparisons, and make more informed decisions, making biplot analysis an essential skill in multivariate analysis interpretation.
Tips for Solving Multivariate Analysis Assignments
Approach multivariate analysis assignments systematically. Thoroughly understand guidelines, select appropriate software for calculations and visualizations, practice with diverse datasets, collaborate for insights, and meticulously document your process. By adhering to these tips, you can confidently navigate the intricacies of your assignments and excel in your multivariate analysis endeavors. Here are some strategies to ace your assignments:
- Understand the Assignment Guidelines: Carefully read the assignment instructions. Identify the goals of the analysis, required techniques, and any specific guidelines provided by your instructor.
- Choose Appropriate Software: Familiarize yourself with software like R, Python (using libraries like NumPy, pandas, and scikit-learn), or specialized statistical software. These tools streamline complex calculations and visualizations.
- Practice with Datasets: Practice on various datasets to gain confidence in applying different techniques. Publicly available datasets or those provided by your instructor can be excellent resources.
- Collaborate and Seek Help: Don't hesitate to collaborate with peers or seek guidance from your instructor or online communities if you encounter challenges.
- Document Your Process: Keep track of your steps, assumptions, and interpretations. This not only helps in understanding your analysis but also in explaining your reasoning in your assignment report.
Conclusion
In the realm of multivariate analysis, a solid grasp of fundamental concepts such as variable types, correlations, and PCA is vital. Selecting the right techniques, like Factor Analysis and Cluster Analysis, empowers you to unveil intricate data relationships. Preprocessing, assumptions validation, and result interpretation are keystones in this journey. By following strategies like software utilization, collaboration, and documentation, you can effectively solve your multivariate analysis assignments. Equipped with these skills, you'll unravel the complexities of multidimensional data and confidently tackle the challenges that come your way.